Avoiding the Pitfalls of AI for Qualitative Data Analysis
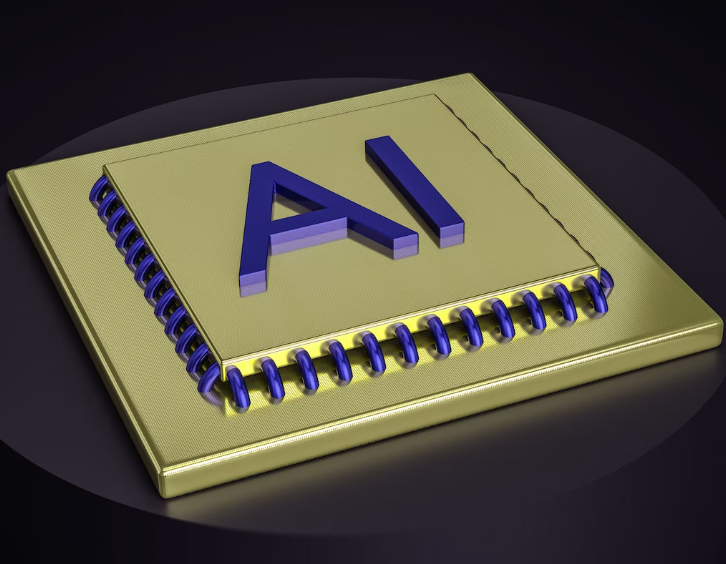
While Artificial Intelligence (AI) is becoming increasingly commonplace in many aspects of our lives, its potential for analysing qualitative data is often overlooked. Qualitative data analysis requires an understanding of context and nuance that AI still struggles with, leading to inaccurate interpretations of the data. Thus, AI is not always the best solution for qualitative data analysis. To ensure data accuracy and avoid mistakes, it is important to understand the pitfalls associated with AI and how to best navigate them.
The first pitfall of AI for qualitative data analysis is its difficulty with context. Qualitative data analysis requires critical thinking, insight, and consideration of the context surrounding the data. AI is only able to analyse the data itself, without taking into account the context and meaning behind it. Thus, interpretations of qualitative data by AI can lead to skewed results and inaccurate conclusions.
A second pitfall of AI for qualitative data analysis is its lack of nuance. Qualitative data analysis often requires fine distinctions, subtle nuances, and creative thinking. AI is unable to pick up on these small nuances and so can only offer basic, basic interpretations. Without a human’s ability to think creatively and synthetically, AI can only offer basic and often inaccurate correlations between data points.
Lastly, AI may be biassed. While AI can process large amounts of data quickly and accurately, it is only as good as the data it is given. Martin Ford’s book “Rise of the Robots” argues that AI is often only as smart as its programmers, meaning the data used to “train” the AI may be flawed or prejudiced. AI can often only interpret data according to its own biases.
Qualitative data analysis is a critical component of decision making. To ensure accurate results and valid interpretations, it’s important to be aware of the pitfalls that come with using AI for this task. AI can be useful for analysing large data sets quickly and accurately but, without an understanding of context and nuances, the conclusions can often be inaccurate or misleading. To avoid mistakes when using AI for qualitative data analysis, it’s important to be aware of the potential pitfalls and take proactive steps to mitigate their effects.
Signup below to get acess to our beta (free for market reserachers to test):